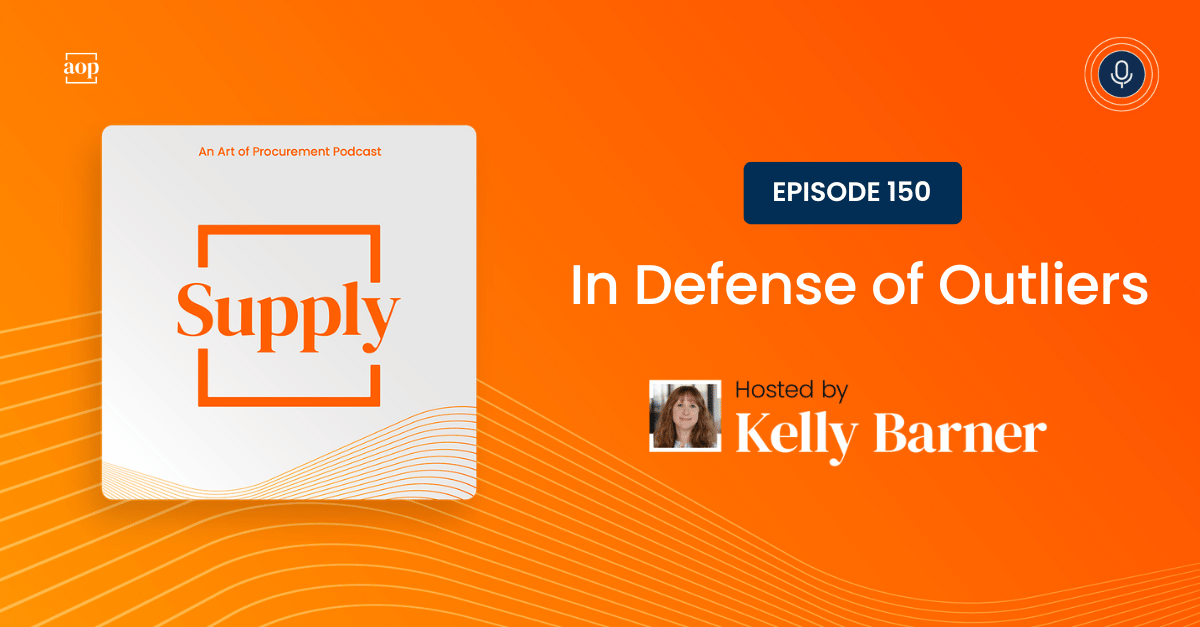
“Polling is a science of estimation, and science has a way of periodically humbling the scientist. So, I’m humbled, yet always willing to learn from unexpected findings.” - J. Ann Selzer, President and Owner, Selzer & Company
On November 1st, 2024, just 4 days before the Presidential election, statistician and FiveThirtyEight founder Nate Silver renewed a charge he has made in the past: pollsters were herding.
Herding is a practice that leads to poll results that very closely resemble each other. Silver’s concern was not that the polls were tight but that there were no outliers. It suggested to him that pollsters were deliberately modeling their polls and baking assumptions into the data to keep them close to each other.
When I read about the practice of herding I thought about two things that seemed worth exploring from a business standpoint:
- There are statistical truths that hold, regardless of how complex an analytical project is
- Human desires and tendencies can skew analytical findings no matter how good our data is
Digging into the background reading for this episode was like people watching - with math.
Inescapable Circular Error
Simply put, polls use sampling to extrapolate information gathered from a small group of people to represent the likely behavior of a larger group of which they are a part.
It is amazing how small of a number of responses can be considered a representative sample. For instance, the median sample size for each swing state poll run in 2024 was around 800. Let’s compare that to the population they are being used to model.
The seven swing states range in population between 3 million (Nevada) and 13 million (Pennsylvania). If we drop Nevada from the list, the other six swing states have populations of between 6 and 13 million. Pollsters have 800 people that they know don’t actually represent 6 or 10 or 13 million people, so they have to make assumptions.
They also know there will be a sampling error - where a non-representative sample is used to model a population. As a result, they weight groups of responses within the 800 to account for population segments by race, economic status, education, political leaning, and likelihood to vote. The assumptions each pollster makes influences their accuracy.
Picking the victor accurately is hard enough but predicting the margin of victory is harder. According to Silver, it is not the potential inaccuracy of polling results that should bother us, but rather the consensus around a wrong result. That consensus is evidence that herding is happening.
For any given poll, the result should be within the margin of error 95 percent of the time and within the standard error 68 percent of the time. According to a Berkley study, official margins of error only cover statistical margins, not any of the other problems with the data or underlying assumptions.
If pollsters are doing their job right, variation is a natural result. Roughly one third of polls should be wrong.
Refuse to Settle for a Comfortable Consensus
Herding reduces the accuracy of polling over time, because it prevents outliers from influencing the movement of the perceived average or center point. If you have enough data, outliers will hardly impact the final finding, but they need to exist and they need to be included.
When herding is happening, it doesn’t matter how many polls there are. If they are all reliant upon each other for their findings, the error will be circular and inescapable.
Over time, in other words as an election approaches, new polls will start to track closer and closer to polling averages. Each pollster wants their final poll to be as close as possible to what actually happens, and the wisdom of the crowd is given a lot of credit. Unfortunately, because that ‘wisdom’ has been baked into the findings multiple times by then, the poll findings have almost nothing to do with reality.
Commentator Jacob Weindling, writing for Splinter.com in advance of the 2024 election, acknowledged that he is not a fan of Nate Silver, but agrees with his statements about herding:
“So why would pollsters herd? Simple human nature, really. They operate in a hyper-competitive environment where ratings from sites like FiveThirtyEight really matter to their business, so if one pollster is going out on a limb relative to everyone else and they are wrong, they will be regarded as less credible going forward. If they are bunched up in the middle alongside a hundred other pollsters and they all are wrong, then they are all safe. Hence, herding.”
The concern about this is that we are all subject to human nature. Whether we are calculating savings within procurement, lane efficiency or risk level within supply chain, or something else in the business altogether, we are going to think about how our analysis and recommendations will be received, creating our own inescapable circular error.
Only if we increase our tolerance for outliers and put energy into understanding and discussing embedded weights and assumptions can we ensure that our data and decisions are based on reality rather than a comfortable consensus.