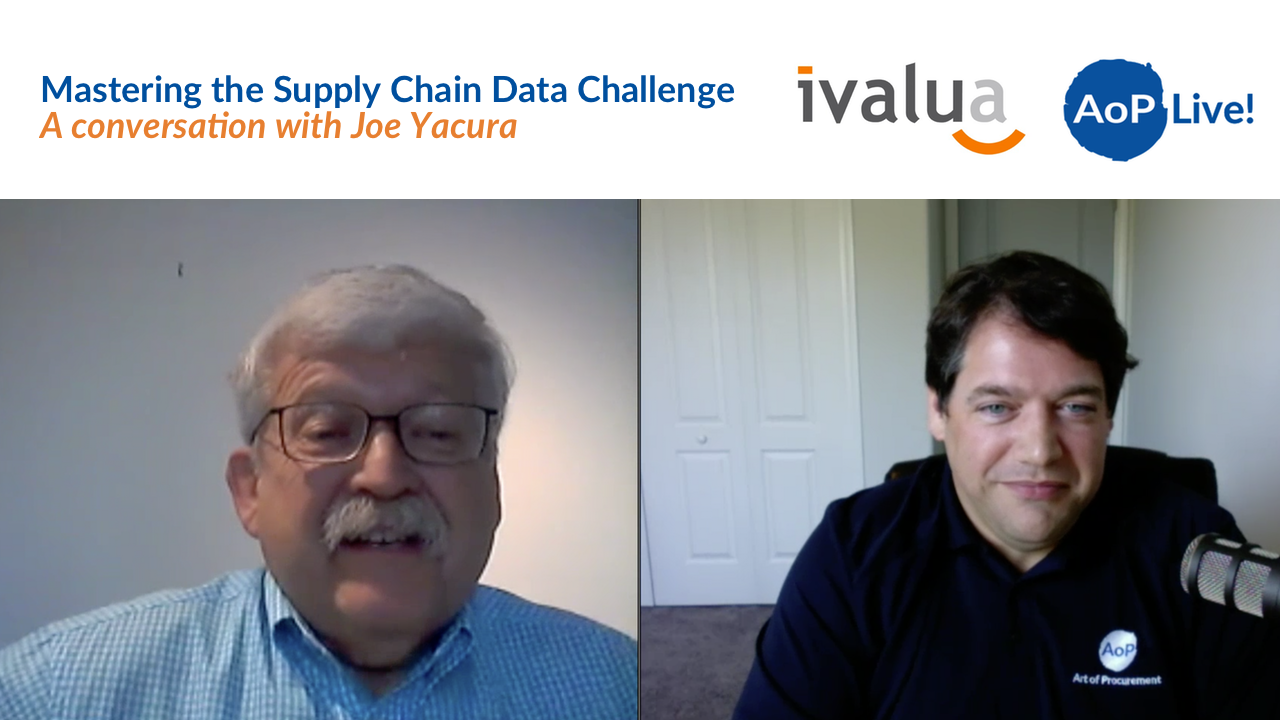
In the latest edition of our AOP Live series, I had the opportunity to speak to Joe Yacura on the importance of data quality – not only as part of procurement digitization, but for the future of procurement as a whole. Joe is a former CPO of organizations such as Fannie Mae, Bank of America and American Express, and the co-author of the 3rd Annual Data Quality and Governance Study in partnership with Dr. Rob Handfield and the Supply Chain Resource Cooperative (SCRC) at NC State.
If it wasn’t apparent before, it should be now: procurement can no longer turn a blind eye to bad data.
We can’t lament but then pass bad data off as a challenge too hard to solve. Procurement has to tackle the problem of bad data quality head-on. The impact of bad data will only be compounded as we increase our reliance on technology to perform the tasks that used to be the domain of the human buyer.
But all hope is not lost!
This challenge is not unique to procurement. Sales, marketing, and quality management teams have long been awash in inaccurate, unstructured, fragmented data. Imagine for a moment the quantity of the information sales and marketing teams have about their target customers. Leading sales and marketing groups have successfully broken down the silos where this data was stored – website traffic, email opens, white paper downloads, phone calls – you name it – into a single source of truth. Doing so has transformed their functions. The same is possible for procurement – and as Joe suggested at AOP Live, we should ask sales and marketing how they did it!
So what do we do first?
Here are some tips that Joe shared with us:
- Undertake a data audit. Understand how fit for purpose your current data is and how it is used.
- Create a data dictionary to be used internally and amongst suppliers to drive consistency in naming conventions.
- Develop a governance plan around data aging. How long will you keep data for? Until what age is data still relevant to your decision-making process?
- Determine what data sources carry more weight than others. Are there sources of data that should be excluded from the decision-making process entirely?
- Formulate a strategy for how often you will review and refresh the algorithms that create your data (i.e. machine learning derived spend analytics). As your data sources change, so must the algorithms that interact with this data.
Over the course of our 45-minutes together, Joe shared a number of insights from the data study about how procurement leaders can mobilize the resource (or resources) necessary to address this data challenge.
So how can procurement get the investment to pay for all of the manual upfront work. Joe shared that most procurement and supply chain executives struggle to address this hurdle. The answer lies in the value attributed to good data vs. bad data. If you can position good data as an asset to your organization – which it undoubtedly is, just ask sales and marketing – and demonstrate the difference in decision making based on a bad data set vs. a good data set, that should be a good place to get this critical but overlooked challenge the attention that it deserves.